This morning, I came across an interesting video by HIMSS TV on a phenomenon called artificial intelligence drift (or AI drift). Reminds me of the Terminator movie series… While many of us in healthcare understand the need for good quality data and the concept of “garbage in, garbage out“, the concept of AI drift is relatively unknown, but is something that many AI engineers and scientists are battling against.
HIMSS video interview with Ronin’s vice president of data science, Dr Christine Swisher. Adopted from MobiHealthNews, 2022.
What is AI drift?
So what is the AI drift phenomenon? Let’s start by defining the term “drift“. “Drift” is usually referred to as a change in distribution over time, and when referenced against computational models, it usually suggests that there is a change or shift in the model’s predictions. As AI becomes more sophisticated, their algorithms start to diverge from their original programmed execution towards activities or responses that are unexpected from their users. The continuous strive towards creating more complex machine learning and deep learning algorithms to something that better mimics human intelligence (e.g. neural networks) has led to a more flexible and collaborative intelligence structure that not just focuses on interpreting data and performing computing tasks, but also towards self-correcting and self-evolving tools.
Are there examples of AI drift?
Movie directors have predicted this phenomenon many years ago. Think about Skynet from the Terminator and the once popular I, Robot starring Will Smith. In these movies, the AI algorithms that were created started to evolve and collaborate with other AI systems in the attempt to destroy mankind and take over, through an evolution of their “way of thinking”. Sounds scary, doesn’t it? Well, you may think that this only happens in the movies, but less than a decade ago, a similar phenomenon happened to Google’s AI translation tool and Facebook’s (now Meta) AI negotiation chatbots, which resulted in these companies shutting down their AI systems!
In September 2016, researchers at Google realized that their Google Neural Machine Translation (GNMT) system was somehow able to automatically translate languages that the system had not been programmed to translate through its own interlingua – an artificial language generated by the AI model between all language pairs involved. Described in their research paper and blog as a “Zero-Shot Translation“, the example provided was that their GNMT system (shown in the animation below) could translate a language pair that was not explicitly programmed, such as Korean⇄Japanese (yellow dotted lines), based on training pairs of Korean⇄English and Japanese⇄English languages (solid blue lines).
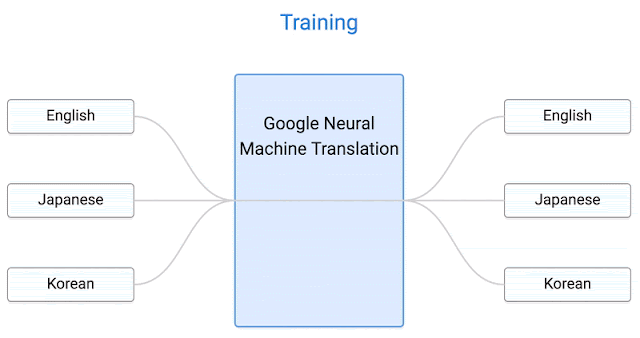
Similarly, in July 2017, Facebook AI Research Lab (FAIR) researchers found that their AI chatbots were able to communicate in a new language to negotiate without human input. In their experiment, their bot was given some items (such as books, hats and balls) and programmed with some preferences for which items it wanted. It then had to negotiate with another party on how to split the “treasures” among themselves. According to their blog post and research paper, there were some interesting and amazing results observed by the researchers:
- The bot had longer conversations when negotiating with humans, which led to it accepting deals less quickly;
- In order to achieve its goals, the bot could initially feign interest in a valueless item, but later compromise by accepting it; and
- The bot could generalize from the training data where necessary in order to produce novel sentences
This kind of sophisticated intelligence may become the norm as AI researchers continue to strive to build machines that can understand consciousness and be self-aware, so as to interact with humans in daily life, just like in I, Robot!
Significance of AI drift in healthcare
AI is becoming a hot topic in healthcare. Every healthcare profession is moving towards AI or putting AI as part of their digital transformation strategy. Application trends in AI can range from AI-based clinical decision support systems, to diagnostic technologies (e.g. artificial pancreas for diabetics) and wearable devices (e.g. OrCam smart glasses for the visually impaired), robot companions (e.g. Pillo and Pria, the pharmacist dispensing robots) and even cyberpharmacy applications in drug discovery and development (e.g. BenevolentAI). Of course, there are many other healthcare applications in the market!
However, one of the concerns that clinicians have is how well the AI model performs in the real-world compared to a controlled research environment. And this is why explainable AI models tend to be preferred over black-box AI models in healthcare. Monitoring for AI drift in AI-based healthcare applications can allow the technical or developer team to easily diagnose issues that may negatively impact the accuracy of AI model, and thus its performance. According to IBM’s AI Blog, there are four challenges that need to be considered:
- Can the AI model be explained?
- Is the AI model unbiased and fair?
- Does the AI model maintain its accuracy over time?
- Can automated documentation be generated from the AI models from data and testing?
The key to minimizing the risk of AI drift in healthcare is to ensure that the AI model is transparent and trustworthy. As such, many healthcare organizations have started to develop their own ethical guidance surrounding AI-based healthcare technologies.
Ethical guidance for AI in health
In order to cater towards a global audience and local audiences to which I am affiliated with in Singapore and Australia, I shall provide a summary of the ethical considerations for AI applications in healthcare. For more details, I encourage international readers to refer to the World Health Organization’s (WHO) Guidance on Ethics and Governance of Artificial Intelligence for Health, and for local audiences, Singapore’s guidance on Artificial Intelligence in Healthcare and the Roadmap for Artificial Intelligence in Healthcare for Australia by the Australian Alliance for Artificial Intelligence in Healthcare. These guidance documents are largely based on the core principles of medical and bioethics.
According to the WHO guidance, AI technologies in healthcare should:
- Protect the autonomy of humans, so that clinicians still have control over the AI technology and any medical decisions generated by the algorithm;
- Promote the well-being, safety and public interest of patients;
- Be transparent, explainable, intelligible and understandable to developers, users and regulators;
- Be used responsibly and accountable by all parties involved in its development, deployment and use;
- Be designed to include its widest possible and equitable use and access; and
- Be able to respond appropriately and adequately to its context of use, and be sustainable throughout its deployment and use.
Outlook of AI in healthcare
In conclusion, while AI currently exists in various technologies that work independently to improve various aspects of clinical workflows and patient care, the real value lies in the integration of all these technologies across the whole patient care journey. Deloitte US paints several holistic scenarios of patient care journeys aided by AI-based technologies in their perspective paper. They predict that with new entrants in the digital health space, AI technologies will soon become a necessary requirement for healthcare companies and organizations to remain competitive. However, it is crucial that these technologies continue to remain as tools to support clinical practices and not “dehumanize” the patient-practitioner relationship.