I had the luxury to attend the Inaugural Artificial Intelligence (AI) Summit held on 11 November 2022 at the Fullerton Hotel in Singapore. Organized by the SingHealth Duke-NUS (National University of Singapore), this full day event consisted of several main lectures and various interesting fireside sessions focusing on AI issues and considerations in various stakeholder sectors. Below are the key takeaways from the Summit, as well as my thoughts on how Singapore is moving forwards into the AI era.
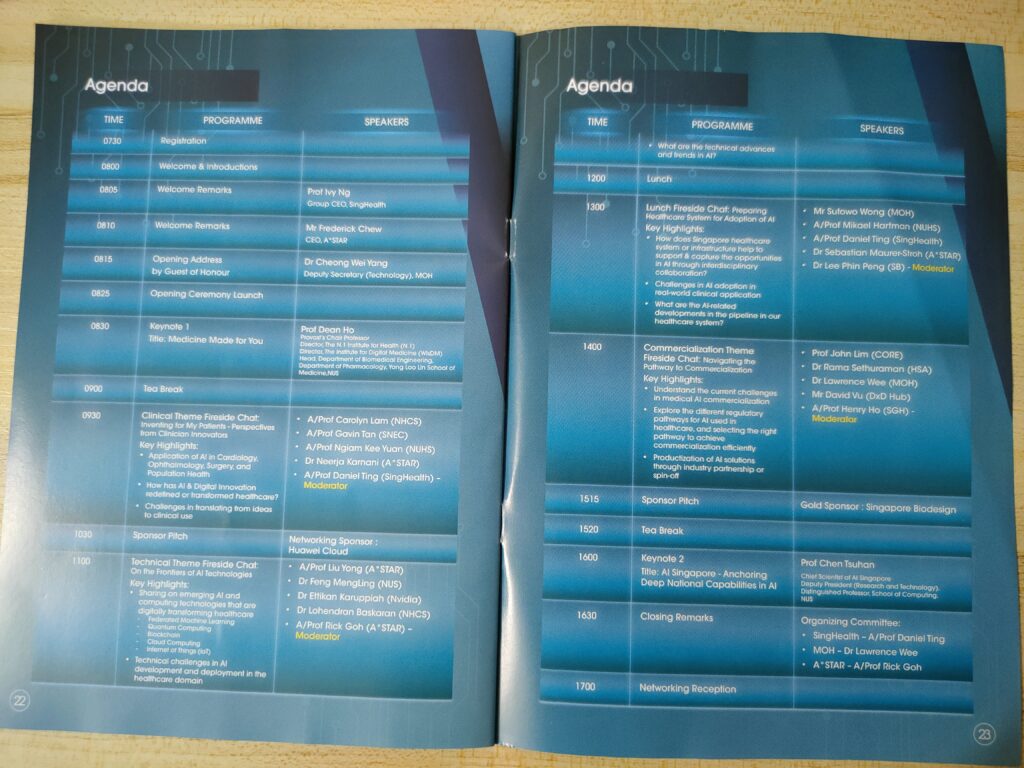
From the 3″B”s to the 3″P”s – Alignment with MOH’s vision
The Guest-of-Honor – Dr Cheong Wei Yang, Deputy Secretary (Technology), Ministry of Health (MOH) Singapore – talked about the need for translatable pathways for AI. As healthcare practitioners, we are definitely familiar with the “3 Beyonds” – Beyond hospital to community, Beyond healthcare to health and Beyond quality to value. He linked the 3 B’s to 3 P’s – referring to Population health, Precision health and Preventive health:
- Beyond healthcare to health –> Precision health
- Of course, precision health in this case is much broader than precision medicine (also known as personalized medicine). With advancements in technology, therapy, health and well-being can be tailored towards the individual, not just a specific group of individuals. According to the US CDC, precision health involves approaches that everyone can do to prevent and treat disease, as well as manage and protect their own health. Examples of such approaches include genome sequencing techniques, genetic testing and counseling, mobile health (mHealth) applications, social media, predictions based on family health histories, biomarkers, and continuous glucose monitoring systems/artificial pancreas, etc.
- Beyond hospital to community –> Population health
- Population health is an approach that aims to improve the health outcomes of a group of individuals or a population through social determinants of health, policies and interventions. Many countries are looking beyond treatment and management of disease in hospitals and considering the paradigm shift towards “hospital-in-a-home“. Singapore is no different – the move towards community-based care is a strategic one that is especially important due to its growing Silver Tsunami population.
- Beyond quality to value –> Preventive health
- Healthcare expenditures are expected to rise in the coming years. As such, preventive health is a key focus in Singapore. By preventing or delaying chronic and severe diseases, Singapore citizens can enjoy a better quality of life and can age more gracefully. With that aim in mind, the Singapore Ministry of Health is developing a Healthier SG initiative to support Singaporeans in improving their health. There are 5 key features to this initiative based on the White Paper passed on 5 October 2022:
- Mobilizing family doctors to deliver preventive care for the public;
- Developing health plans to promote lifestyle adjustments, regular health screenings and appropriate vaccinations;
- Activating community partners (e.g. Health Promotion Board, People’s Association, Sport Singapore) to support the public in leading healthier lifestyles;
- Launching a national enrolment program for residents to see one family doctor and adopt a health plan; and
- Setting up the necessary key enablers (e.g. IT, manpower, financing policy) to make Healthier SG work.
- Healthcare expenditures are expected to rise in the coming years. As such, preventive health is a key focus in Singapore. By preventing or delaying chronic and severe diseases, Singapore citizens can enjoy a better quality of life and can age more gracefully. With that aim in mind, the Singapore Ministry of Health is developing a Healthier SG initiative to support Singaporeans in improving their health. There are 5 key features to this initiative based on the White Paper passed on 5 October 2022:
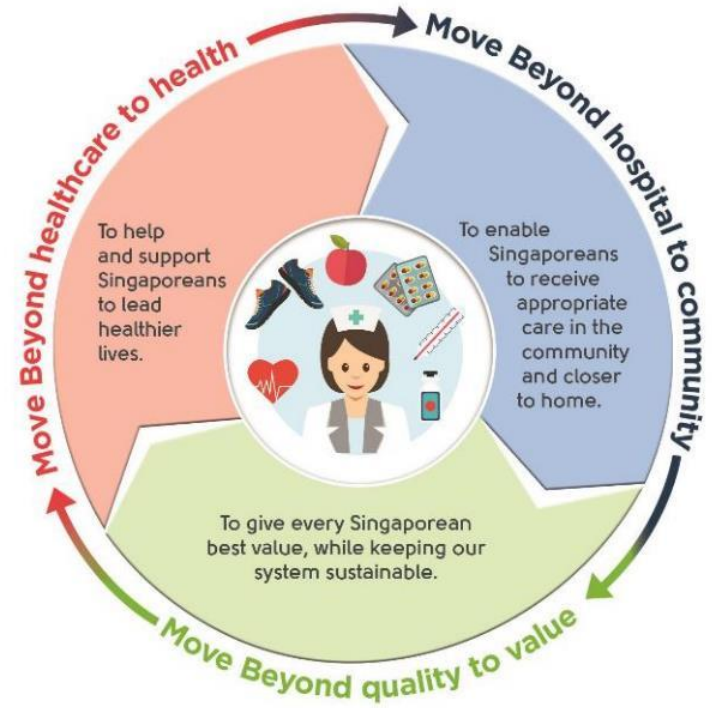
Strategic focus on AI
It was acknowledged in the Summit that there is a need to understand the AI algorithms better if we were to leverage AI for healthcare as a country. This requires synergy in terms of collaborations between clinicians and AI specialists. For example, a clinical specialist can work with AI specialists/scientists to generate different models for different patient populations. If the AI algorithms can be understood and interpreted (i.e. explainable AI) well enough to stratify the points and segment the population, we do not need to sacrifice specificity for sensitivity, and can identify which AI model(s) will be the best for the different populations.
As such, A*Star (Agency for Science, Technology and Research) has started to focus on 4 key domains in AI to balance the risks and potential of AI in healthcare. These are:
- Responsible AI – According to Accenture, responsible AI is the practice of designing, developing and implementing AI with good intentions, so as to empower and fairly impact users and consumers, allowing trust and enabling the scaling of the AI with confidence. In healthcare, there are 6 key characteristics that are important in understanding responsible AI – accountability, empathy, fairness, transparency, trustworthiness and privacy.
- Rationalizable AI – The concept is the same as “explainable AI“, in which the AI algorithms should be able to be interpreted/logically explained in order to gain a greater acceptance among various stakeholders in the healthcare sector.
- Sustainable AI – A movement to foster a change in the lifecycle of AI products towards greater ecological integrity and social justice. In view of developing AI that is compatible with sustaining environmental resources, there are two main branches of focus: AI for sustainability and sustainability of AI. As such, sustainable AI should not only be applied to AI applications, but the broader AI ecosystem.
- Synergistic AI – This domain focuses on how AI applications can work in close collaboration with other fields (e.g. big data, additive manufacturing and clinical expertise) to bring about a greater understanding towards diagnosis, treatment and management of diseases.
Ethics and governance of AI
Another topic that is gaining attention worldwide (including Singapore) is the ethics and governance of AI. Based on the traditional principles of bioethics, the ethical principles of AI includes various clusters regarding the transparency, justice and fairness, non-maleficence, responsibility, privacy, beneficence, freedom and autonomy, trust, sustainability, dignity, and solidarity of AI. Many countries are developing their own national policies and regulatory frameworks to ensure that AI is trustworthy and responsible for healthcare. In fact, the UNESCO Member States had adopted a global agreement on the ethics of AI in November 2021. Closer to home, MOH, in collaboration with Integrated Health Information Systems (IHiS) and the Health Sciences Authority (HSA), also developed a set of recommendations to encourage the safe development and implementation of AI-based medical devices in 2021. Known as the Artificial Intelligence In Healthcare Guidelines (AIHGle), its objective is to share good practices with developers and implementers of AI in Singapore’s healthcare system, as well as to complement HSA’s current regulations of medical devices. A summary of the guidelines is appended below.
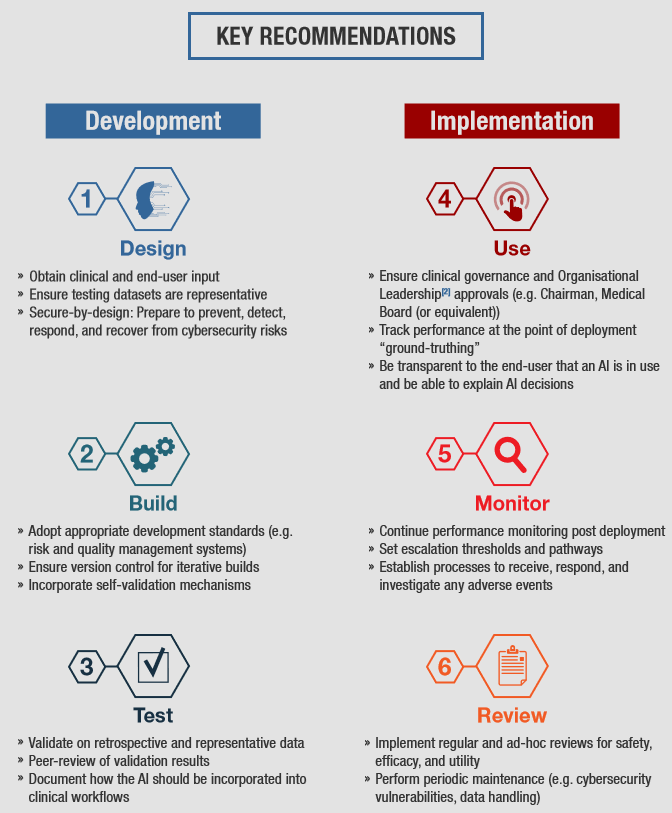
The topic on the legality and responsibility of wrong AI decision-making was also briefly touched upon. In Singapore, the responsibility clearly lies with the decision maker, i.e. he/she will be the one who is legally responsible for any consequences resulting from an AI model. The current legislation is still very much based on the patient-practitioner relationship, not the patient-company relationship. Therefore, the clinician takes the responsibility of whatever decision that they make, regardless of whether it is with or without any AI assistance.
In one of the fireside chats, it was also discussed on whether AI needs to be 100% explainable in order for clinicians to be able to trust the algorithm. An analogy was provided by one of the panel members, in terms of a drug treatment. In many cases, a clinician does not need to know fully the mechanism of action of a drug in order to trust that it will be beneficial to the patient. In fact, the impetus to use a drug for particular conditions is because of its strong evidence of efficacy in the medical condition. The same should be said of AI – as long as there is strong and robust evidence that an AI works, one does not need to fully understand how the algorithm works in order to use it for clinical purposes.
Preserving privacy of AI-based health data
Of course, any discussions on AI will always touch on the topic of the privacy and confidentiality of health-related data. To this extent, there has been much research on how to preserve the privacy of health-related data in AI systems. The purpose of privacy-preserving systems is to allow AI models to work with as minimal personal data as possible, yet not compromising on its functionality. A local example is at the Nanyang Technological University (NTU), where they have set up the Strategic Centre for Research in Privacy-Preserving Technologies & Systems (SCRiPTS), which focuses on the research, development and application of privacy-preserving techniques that are aligned to Singapore’s national priorities. In fact, the UK’s Information Commissioner’s Office (ICO) has neatly summarized some of the privacy-preserving techniques in their blog:
- Minimizing personal data for training:
- There are several feature selection methods that can be used by data scientists to select certain features in a dataset that will be useful for training the AI model.
- Certain data points belonging to individuals can be changed in a way by “adding noise” to the data, yet preserves the required statistical properties of the features.
- Another relatively new method of preserving privacy is federated learning. This process allows multiple parties to train AI models on their own data, and then combine their models with other models into a single, more accurate “global” model. The advantage of this method is that the individual parties do not need to share their training data with each other. This method is envisioned to have several large scale applications.
- Minimizing personal data for inference:
- Personal data can be converted into less human-readable formats (i.e. more abstract formats) so that the data is less easily recognized.
- Personal data can be hosted locally on the device that collects and stores the data, rather than being hosted on the cloud.
- Other privacy-preserving query approaches exist to minimize the data that is sent to the machine learning model, so that the party running the AI model can still perform the prediction/classification without the need for personal data.
- Anonymizing data:
- Certain types of personal data can be made anonymous or pseudonymous in some cases, so that it can be fed to machine learning systems with lesser risks of identification.
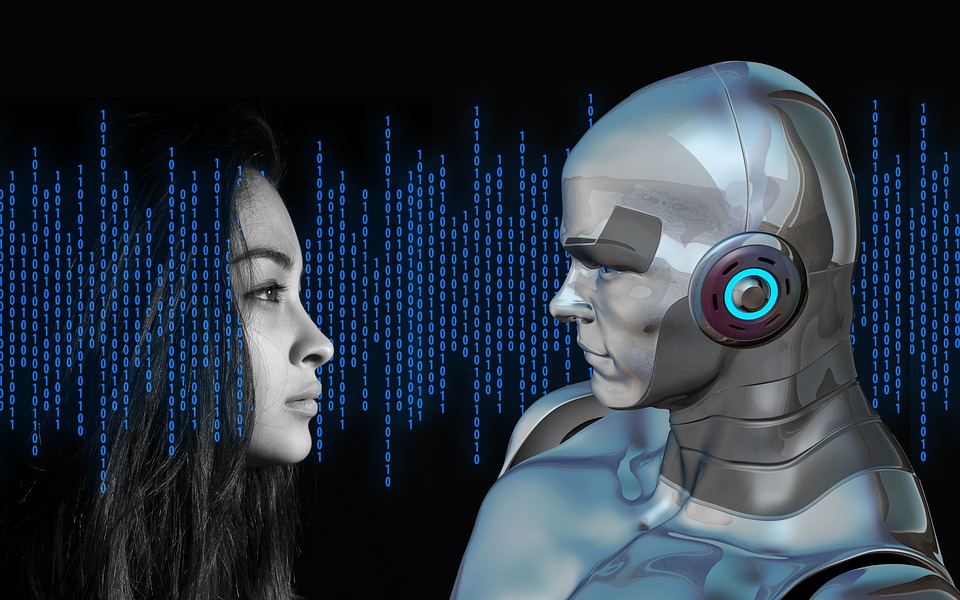
Big data is not always good for prediction
Although “big data” is the buzzword right now, for purposes of healthcare and the risks of identification based on personal data, big data is not always the best. In fact, the notion of “small data” or data that is “just right” is the direction to go for machine learning and deep learning in healthcare.
Professor Dean Ho, Director of WisDM (N.1 Institute for Health, Institute of Digital Medicine, NUS Yong Loo Lin School of Medicine), provided an interesting lecture on how his team has managed to use the patient’s own data to do personalized prediction of their disease conditions, so that clinicians can be better informed on how to treat and manage the patient. The platform, known as CURATE.AI, is an AI-based small data technology platform for personalized dosing of medicines. Using small data collected exclusively from the patient, CURATE.AI uses a quadratic equation to generate an individualized profile of the patient, as well as recommend dosing. The team has published many papers on the platform’s applications in metastatic cancer, hypertension and diabetes, among others. Another similar platform was also recently developed by the team, called IDentif.AI, to help with optimizing combination therapy for COVID-19 and other infectious diseases.
Will AI replace healthcare professionals?
Last but not least, the Summit addressed an inherent question that had been lingering in our minds for the longest time – “Will AI replace healthcare professionals?” This notion has been asked by many healthcare professionals since the dawn of AI, and there have been controversies on this topic for various professions. The takeaway that I got was that while AI can replace the most mundane functions that are currently being performed by healthcare professionals, at its current state, it will not be able to replace us. In fact, a new perspective was brought up – that AI will instead make doctors/healthcare professionals “immortal”! An AI model needs data to be input for it to be accurate and sensitive. Healthcare professionals will be the ones to provide these data and insights into the AI models, so that the AI systems can work as intended. With time, the younger generations of healthcare professionals will continue to work with these AI systems in accordance to their change and updates in practices – to better the models, which in turn will improve and grow the AI ecosystem at a faster pace. Our wealth of knowledge, experience and wisdom will ultimately be embedded in these systems in time to come, in a sort of computational “genetic code” for the future! Nonetheless, it was agreed by attendees of the Summit that AI should only be an assistive/augmentative agent, but not replace jobs, at least in healthcare.
All-in-all, this was another interesting conference that provided lots of food for thought. I will definitely be excited to attend next year’s conference!